Why Sumo Logic
Meet the world’s most powerful
and scalable log analytics platform
01
One integrated log analytics platform
A single source of truth for development, security and operations teams.
02
Cloud-native, distributed architecture
Scalable, multi-tenant platform purpose-built for structured and unstructured logs – never drop your data.
03
$0 ingest and credit licensing
Flex Licensing decouples log ingest from budgets, eliminating data gaps during troubleshooting.
04
Machine learning and advanced
analytics
Identify, investigate, and resolve issues faster and more accurately with AI/ML, including natural language search with Mo Copilot.
05
Secure by design
Multi-million annual investments on regulatory compliance certifications, attestations, pen testing, code reviews and bug bounty programs.
06
Faster incident response
Add context to security alerts with threat intelligence data and respond to issues faster with customizable, automated playbooks.
How it works
Ingest everything. Dashboard everything. Query everything. Overlook nothing.
How teams like yours use Sumo Logic to turn complexity into insights.
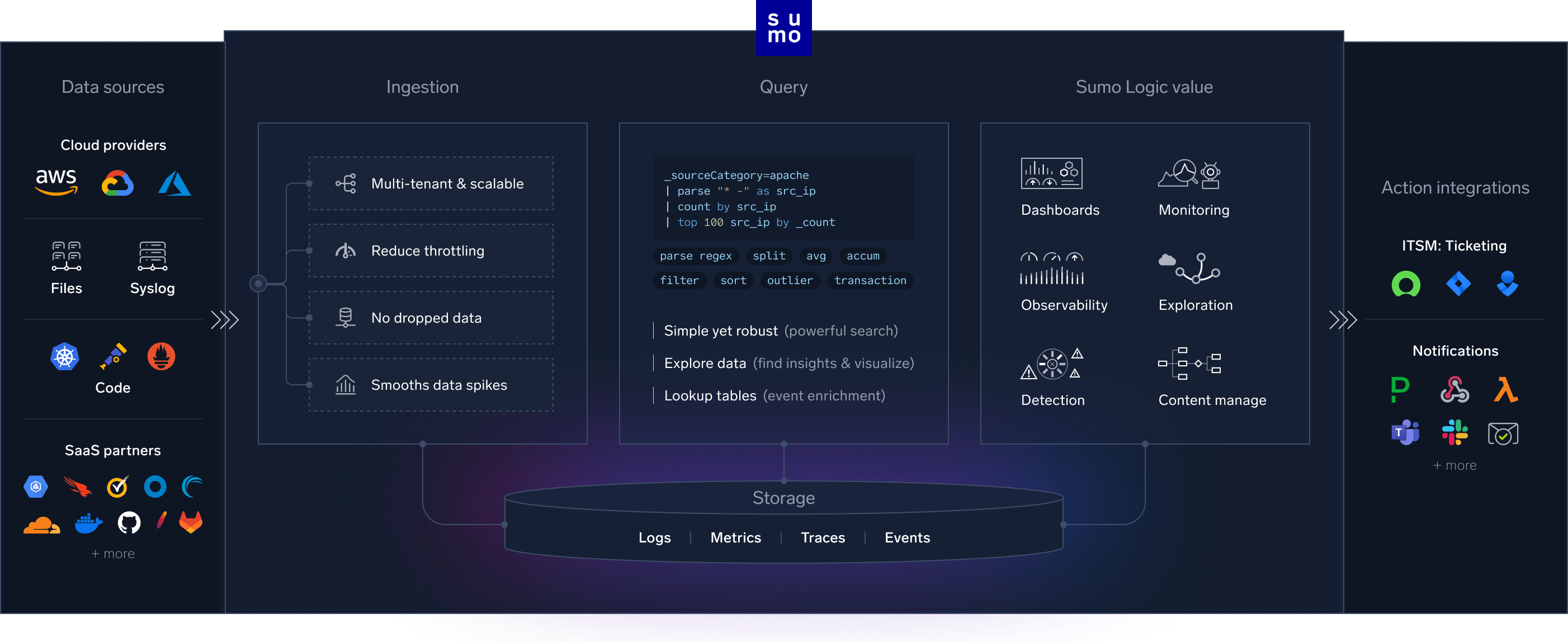
Solutions
Transform complexity into insights
Log Analytics
AI-powered troubleshooting and out-of-the-box dashboards
Application Observability
Infrastructure Monitoring
Logs for Security
Compliance and Audit
Cloud SIEM
Getting started
Find the needle in a haystack —
using powerful log analytics
01
Start free trial
Get up and running in minutes. No credit card required.
- 30-day full feature trial
- No credit card required
- Deploy in minutes
02
Integrate your data
Easily collect structured and unstructured logs with 100s of OOTB integrations.
- Integrate with SaaS vendors
- Kubernetes, AWS, logs and metrics
- Full OpenTelemetry collections
03
See it in action
Prebuilt, customizable queries, dashboards, monitors and alerts.
- Explore data
- Create your dashboards
- Create monitoring and alerts
Resources
Resources at your fingertips
FAQ
Still have questions?
Sumo Logic helps you monitor, troubleshoot and secure your applications with a single SaaS analytics platform.
- One SaaS analytics platform for observability and security
- Cloud-native architecture — dynamic scale
- Patented ML-based analytics — ingest and analyze any type of data fast
- Zero dollar log ingest eliminates data gaps during troubleshooting and root cause analysis
- Out-of-the-box audit and compliance, including PCI DSS, FedRAMP Moderate, HIPAA, SOC 2 Type 2
It’s easy to sign up. Register with your email to start your 30 day free trial—no credit card required! Check out our quickstart guide to learn about Sumo Logic and get up and running in minutes.
Collect logs using our hundreds of out-of-the-box integrations, including:
- Cloud providers AWS, Azure, and GCP
- Containers, such as Kubernetes and Docker
- Database servers Oracle, MongoDB, etc.
- Web-based servers like Apache and NGINX
- Security applications, including Okta and Zacaler
- Productivity tools, such as Salesforce, Jira and Zoom
Sumo Logic has three different types of data collectors:
- Hosted Collectors reside in the Cloud for seamless connection to cloud services
- Installed Collectors are installed on a local machine.
- OpenTelemetry Distribution is an installed agent for all data built entirely with opentelemetry-collector-builder, providing a single unified agent to send logs, metrics, traces and metadata to Sumo Logic.
Share dashboards with specific users, roles, or your entire organization. Role-based access control (RBAC) and security policies allow you to control the data access level of users.
Find and isolate issues in Sumo Logic — from alerting to identifying anomalies to performing root cause analysis. Our Search Query Language, coupled with machine-learning features like LogCompare, Time Compare, LogReduce and LogExplain helps you extract insights from your infrastructure and application data.
See Get Started with Search to learn more.
No, Sumo Logic operates zero-dollar ingest licensing (called “Flex”) that is aligned to the amount of log data you query in the platform. There are no restrictions on users accessing Sumo Logic, except for advanced security management features such as Cloud SOAR, which are subject to incremental qualifications. More on our Flex Licensing model and packages can be found on our pricing page.
Sumo Logic applies best-in-class technologies and a rigorous process to put the safety of your data first, including encryption-at-rest and security attestations. Compliance attestations and certifications held by Sumo Logic include PCI, HIPAA, FISMA, SOC2, GDPR and FedRAMP — at no additional charge.
Sumo Logic’s flexible credit-based pricing helps customers deliver secure and reliable applications. Whether you start a trial or adopt our Enterprise Flex package, we’ll give you the flexibility to manage your data without any surprises.
Zero-dollar ingest lets you ingest all your log data, only charging you for the insights you derive by querying the platform. Maximize your analytics and eliminate budget waste by paying for the greatest value you receive.